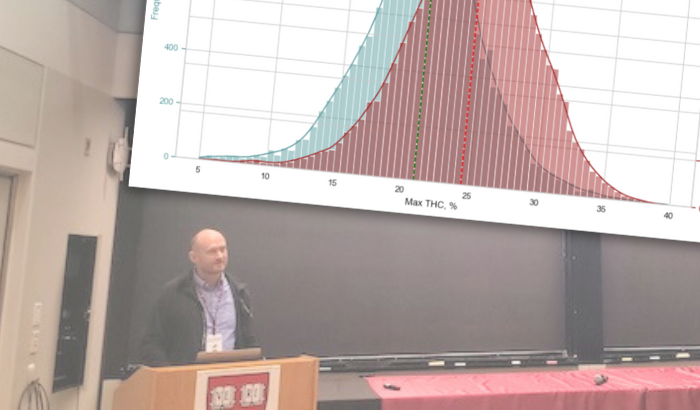
“I won’t say which lab is manipulating data and which isn’t, but both can’t be right.”
For a new market and industry as exciting as cannabis, it may seem disappointing that the biggest story of the year is about data.
But in this case, that data relates to product potency and billions of dollars worth of potentially mislabeled products, so it essentially affects just about everyone and everything, from testing labs and cultivators to customers, who often make their purchasing decisions based on THC percentage.
Is this a scandal? To put it simply, yes, and an enormous one that is unfolding in multiple states, including in Massachusetts. With a current lack of strong enforcement around potency-measuring regulations, there’s no telling how severely buyers are being taken advantage of.
Furthermore, the question of widespread testing manipulation is germane to headline-grabbing power struggles at the Cannabis Control Commission, where reported disagreements over how to manage the potency issue have come up around public agency disagreements and the suspension of Chair Shannon O’Brien. Last month, the CCC itself began to address the topic, issuing a bulletin to standardize cannabis testing that watchdogs say is a promising but small start.
As we have reported over the past several months, a handful of scientists and consumer advocates have taken on the task of getting to the bottom of the problem and pushing for change. One of them is Yasha Kahn, the VP of marketing and technology at MCR Labs in Framingham. As he put it, “If ExxonMobil was watering its gas down by 20%, you’d hear about it, and attorneys general would speak up and get the dollar signs in their eyes.” But with weed, it’s different.
Kahn has spent the past year-plus compiling raw testing data from multiple states, and presented his findings in public for the first time in a keynote address at the Cannabis Science Fair at the Harvard Science Center this past weekend. Transcribed and edited sections from his lecture can be found below …
Collecting the data …
Through a bunch of Freedom Of Information Act requests, we gathered data sets from multiple states, and there was one state that already had open data. Together it’s across five states [including Massachusetts], 80 labs, over a number of years, and it has 600,000 flower samples. So it’s a whole lot of data. And now all of that data is fully available to everyone.
Cleaning the data …
Different states have different regulations, so we needed to normalize the data across the states. Then we normalize across the different regulations and we moisture adjust since some states prohibit moisture adjusting and some states mandate it. We wanted to make sure that we can compare all labs and all results across the country.
Once we did that, we had this huge data set and we needed to understand the integrity of the data. There are some truths: most lab potency data sets exhibit normal distribution, while some labs exhibit statistical anomalies; and THC measurements across 28 labs, excluding small and anomalous data sets, had mean THC averages of 21.67%. Most labs have pretty much the same average potency.

The problem at hand …
In cannabis, there are incentives [to inflating potency numbers], and we know what they are—[the relationship between] the amount of THC in a product and price. Flower with under 20% THC typically doesn’t sell, and the more THC that’s in a product, the more it can be sold for and the faster it can sell.
[Looking at data from specific labs] that are aware of this pricing scheme… this one lab I assume has a model internally to not report anything under 20%. … But another lab, in another state, has exactly the same scheme. Because growers tell labs, we will leave you if you don’t do this.
Subjectively, I see that there’s a discontinuity in all of these [data sets], and that there’s something happening at [the 20% THC mark]. … There are tools that are used by courts and in other places that can answer the question of, could this have occurred through randomness? And they all result in, yes we need to take a closer look.
How manipulation happens …
Such labs are found in every state, but these labs don’t necessarily inflate every sample. They take the samples that do not fit the narrative and they seem to manipulate those. The way that they can do this, from the most expensive to the least expensive approach, is: to retest until the desired result is attained, but that takes way too long and is way too expensive; easier is to manipulate the second test, if you know that it’s at 14% instead of 20%; and even less expensive is to manipulate the result of the first test—you see that it’s at 18%, and you write that it’s at 20.1%. Labs have been caught doing this, labs have been fined for doing this. And the least expensive [way to manipulate] is a dry lab, with fake tests. Why even test it if you’re going to fake the numbers?
It is systematic manipulation when they use the same kind of approach for all samples. This can be done by everyone in the lab who is aware of it—an example is, when testing flowers, you put as many trichomes in [the sample] as you can. Or the most egregious is you take a sample, put it in a container, shake it up, and whatever falls off, you test. And you will get significantly higher potency results. Or there is the lone-wolf approach, in which a single person in the lab knows that this is happening, and they manipulate the instruments or the calculations.
Identifying bad actors …
For the most part, labs can be divided into three groups—those with major discontinuities [in their potency data], those that have the average Max THC of around 19 and a half to 21%, and those who have a Max THC of over 24%. Try to align [the data for all of these labs], and they don’t align.
With a data set of this size, what we can do is identify growers that have sent the same sample to multiple labs. We know that it’s the same sample because of the names, and they’re sent around the same time. From that, we get two different data sets, and we run something called the Student’s t-test, a statistical tool with which we can ask the question of whether two different data sets have been created through randomness, or if something else has happened. Randomness could not have caused such a difference, the conclusion is that the approach to measurement is different.
The allegory …
Let’s say you’re a man that’s 5 ft 9 in and someone comes to town and says, Hey, test your height with me, and tells this man who is 5 ft 9 in that [he is] 6 ft 10 in now. I don’t think that people are going to believe the guy saying it—we know it’s not true. I won’t say which lab is manipulating data and which isn’t, but both can’t be right.
On year-over-year increases in THC levels on product labels …
We see growth in potency [across states] every year. And that’s expected, because growers know that flowers under 20% THC don’t sell. But the real reason that we see that in the data is that the labs that offer higher potency get more business. Every state has dishonest labs, and when a single lab shows up and offers to a single grower service with such impossible results, every other grower in the state, in order to compete, needs to make the decision—do we go out of business, or do we inflate? And every lab has to do the same thing every time a grower comes to a lab and says, but that other lab is offering higher potency. The lab has to decide if they start to manipulate potency or if they go out of business. Over the last month. I know of two honest labs that have gone out of business. There are states without a single honest lab left.
The extent of the damage …
I estimate that between 1 and 2 billion dollars in mislabeled flower is sold per month in the US. And flower is just the first product that’s made. Because from mislabeled products, someone has to make concentrates, and from that they make edibles.

Possible solutions …
Kahn’s notes on things that have worked in other states to address the potency manipulation problem:
- Make compliance testing data publicly available.
- Conduct transparent off the shelf testing.
- Penalize the behavior of fraudulent labeling and data reporting, including recalling products when off-the-shelf testing shows major discrepancies, suspending and fining operators that systemically mislabel products, and informing operators that audits will be conducted, and bad operators will face consequences.